Web Scraping with LangChain and ProxyTee: A Step-by-Step Guide
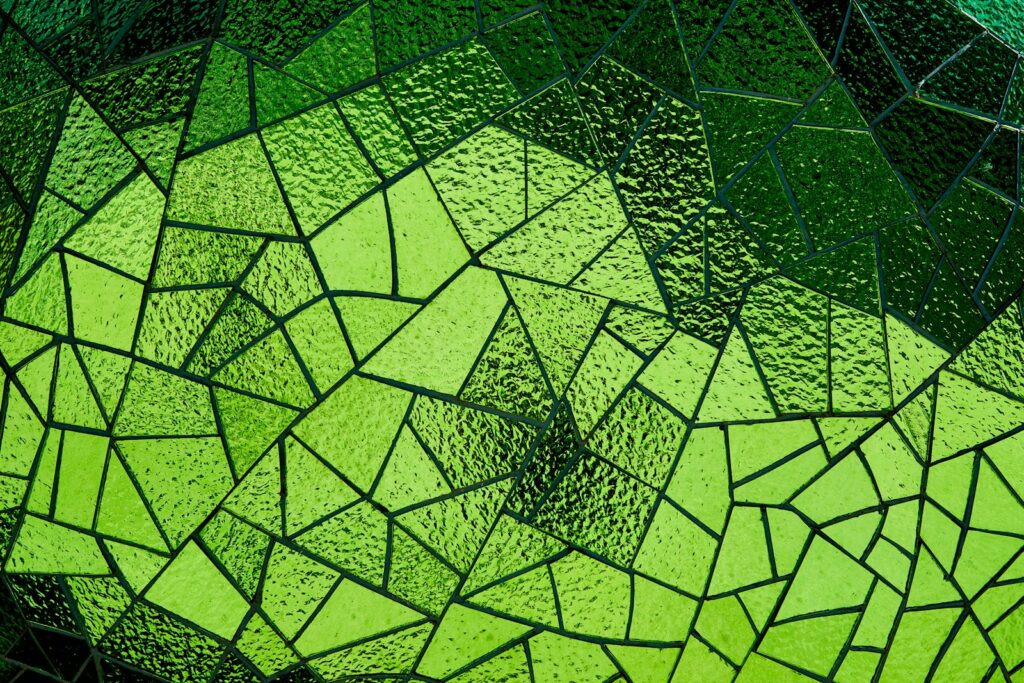
In this guide, you’ll learn how to combine web scraping with LangChain for real-world LLM data enrichment, using ProxyTee. Discover how ProxyTee’s residential proxies make this process seamless and efficient. This will empower you to use web scraping to power your LLM applications. Let’s dive in!
Using Web Scraping to Power Your LLM Applications
Web scraping involves retrieving data from web pages, which can then be used to fuel Retrieval-Augmented Generation (RAG) applications and leverage Large Language Models (LLMs).
RAG applications require access to real-time, domain-specific, or expansive datasets that might not be available in static databases. Web scraping bridges this gap by extracting structured and unstructured data from various web sources, such as articles, product listings, or social media.
Benefits and Challenges of Using Scraped Data in LangChain
LangChain is a powerful framework for building AI-driven workflows. It enables the seamless integration of LLMs with various data sources. It excels at data analysis, summarization, and question-answering by combining LLMs with real-time, domain-specific knowledge. However, acquiring high-quality data remains a challenge.
Web scraping can address this issue but also introduces challenges, such as anti-bot measures, CAPTCHAs, and dynamic websites. Maintaining compliant and efficient scrapers can be time-consuming and technically complex. This is where ProxyTee comes in.
ProxyTee offers a range of residential proxies designed to handle these challenges. With features like unlimited bandwidth, a global IP pool, and auto-rotation, ProxyTee ensures that you can scrape data efficiently without encountering blocks or CAPTCHAs.
With ProxyTee’s advanced features such as IP rotation and broad global coverage, data collection becomes reliable and hassle-free. Plus, its simple API integration allows developers to integrate seamlessly with various applications and workflows, ideal for businesses seeking to automate their tasks.
LangChain Web Scraping Powered By ProxyTee: Step-by-Step Guide
In this section, we will guide you through building a LangChain web scraping script using ProxyTee. We will retrieve content from a CNN article using ProxyTee’s residential proxies, and then send it to OpenAI for summarization using LangChain.
We will target the following CNN article:
This example will provide a simple starting point, which can be easily extended with additional features and analyses using LangChain. For instance, you could create a RAG chatbot based on collected data.
📝 Prerequisites
To follow this tutorial, you will need:
If you are missing any of these, do not worry; we will guide you through the entire process.
Step 1️⃣: Project Setup
First, verify that you have Python 3 installed. If not, download and install it.
Open a terminal and run the following command to create a folder for your project:
mkdir langchain_scraping
Navigate to the project folder and initialize a Python virtual environment:
cd langchain_scraping
python3 -m venv env
Note: On Windows, use python instead of python3.
Open the project in your preferred Python IDE, such as PyCharm or Visual Studio Code. Create a script.py file inside the project. Then activate your virtual environment in the IDE’s terminal by running the command below:
./env/bin/activate
or, on Windows:
env/Scripts/activate
Step 2️⃣: Install the Required Libraries
Your LangChain web scraping project requires these libraries:
- python-dotenv: Loads environment variables from a .env file, used to manage sensitive information like API keys.
- requests: Performs HTTP requests to interact with web scraping endpoints.
- langchain-community: LangChain integrations.
- langchain-openai: LangChain integrations for OpenAI through its openai SDK.
Install these dependencies using pip:
pip install python-dotenv requests langchain-community langchain-openai
Step 3️⃣: Prepare Your Project
Add the following imports to script.py:
from dotenv import load_dotenv
import os
Then, create a .env file in your project folder. This file will store your credentials.
Load the environment variables from .env using:
load_dotenv()
You can now read environment variables from .env files using:
os.environ.get("<ENV_NAME>")
Step 4️⃣: Configure ProxyTee
As noted earlier, web scraping has several challenges. Luckily, it’s significantly easier with a comprehensive solution like ProxyTee. ProxyTee’s Unlimited Residential Proxies provides access to over 20 million IPs across 100+ countries.
To get started, sign up for a ProxyTee account. You will receive credentials to access your proxies. Store these credentials in your .env file as follows:
MYPROXY_USERNAME="YOUR_MYPROXY_USERNAME"
MYPROXY_PASSWORD="YOUR_MYPROXY_PASSWORD"
Step 5️⃣: Use ProxyTee for Web Scraping
Here’s an overview of how you can use ProxyTee’s residential proxies for scraping:
First, configure ProxyTee’s proxy server in your Python script by reading credentials from your .env and define the proxy settings:
MYPROXY_USERNAME = os.environ.get("MYPROXY_USERNAME")
MYPROXY_PASSWORD = os.environ.get("MYPROXY_PASSWORD")
proxy_host = "us.proxytee.com"
proxy_port = 12345 # Replace with your desired port
proxies = {
"http": f"http://{MYPROXY_USERNAME}:{MYPROXY_PASSWORD}@{proxy_host}:{proxy_port}",
"https": f"https://{MYPROXY_USERNAME}:{MYPROXY_PASSWORD}@{proxy_host}:{proxy_port}",
}
Now, modify your get_scraped_data function to use these proxy settings when sending the HTTP requests. Add a proxy argument in the function for easier testing:
def get_scraped_data(url, proxy=None):
response = requests.get(url, proxies=proxy)
if response.status_code == 200:
# You might need to parse the HTML using Beautiful Soup or similar tools
# here for complex web pages, but this CNN scraper works without it
return response.text
else:
print(f"Error: {response.status_code}")
print(response.text)
return None
The function uses the proxies settings and send a get request to the provided url
Step 6️⃣: Get Ready to Use OpenAI Models
For LLM integration with LangChain, this example relies on OpenAI models. You need to configure an OpenAI API key as an environment variable. By default, langchain_openai automatically reads the API key from the OPENAI_API_KEY environment variable.
Add the following line to your .env file, replacing with your OpenAI API key:
OPENAI_API_KEY="<YOUR_OPEN_API_KEY>"
Step 7️⃣: Generate the LLM Prompt
Create a function that generates a prompt based on the scraped data, requesting a summary of the article. The default prompt will use 100 words, however the words number can be modified by passing another value.
def create_summary_prompt(content, words=100):
return f"""Summarize the following content in less than {words} words.\n\n CONTENT:\n '{content}'\n """
Step 8️⃣: Integrate OpenAI
Now, retrieve content from the CNN article and configure the ChatOpenAI object using the ProxyTee configured proxy:
article_url = "https://www.cnn.com/2025/12/16/weather/white-christmas-forecast-climate/"
print(f"Scraping data from '{article_url}'...")
scraped_data = get_scraped_data(article_url, proxy=proxies)
if scraped_data is not None:
print("Data successfully scraped, creating summary prompt")
prompt = create_summary_prompt(scraped_data)
print("Sending prompt to ChatGPT for summarization")
model = ChatOpenAI(model="gpt-4o-mini")
response = model.invoke(prompt)
summary = response.content
print("Received summary from ChatGPT")
Make sure you import the ChatOpenAI module from the library langchain_openai as below:
from langchain_openai import ChatOpenAI
Step 9️⃣: Export the AI-Processed Data
Finally, export the data processed by the AI model into a JSON file.
export_data = {
"url": article_url,
"summary": summary
}
file_name = "summary.json"
with open(file_name, "w") as file:
json.dump(export_data, file, indent=4)
print(f"Data exported to '{file_name}'")
Remember to import json:
import json
Step 🔟: Add Some Logs
To track the script’s progress, you can include print statements at key steps in the script.
📰 Put It All Together
Your final script.py file should contain:
from dotenv import load_dotenv
import os
import requests
import json
from langchain_openai import ChatOpenAI
load_dotenv()
MYPROXY_USERNAME = os.environ.get("MYPROXY_USERNAME")
MYPROXY_PASSWORD = os.environ.get("MYPROXY_PASSWORD")
proxy_host = "us.proxytee.com"
proxy_port = 12345 # Replace with your desired port
proxies = {
"http": f"http://{MYPROXY_USERNAME}:{MYPROXY_PASSWORD}@{proxy_host}:{proxy_port}",
"https": f"https://{MYPROXY_USERNAME}:{MYPROXY_PASSWORD}@{proxy_host}:{proxy_port}",
}
def get_scraped_data(url, proxy=None):
response = requests.get(url, proxies=proxy)
if response.status_code == 200:
# You might need to parse the HTML using Beautiful Soup or similar tools
# here for complex web pages, but this CNN scraper works without it
return response.text
else:
print(f"Error: {response.status_code}")
print(response.text)
return None
def create_summary_prompt(content, words=100):
return f"""Summarize the following content in less than {words} words.\n\n CONTENT:\n '{content}'\n """
article_url = "https://www.cnn.com/2025/12/16/weather/white-christmas-forecast-climate/"
print(f"Scraping data from '{article_url}'...")
scraped_data = get_scraped_data(article_url, proxy=proxies)
if scraped_data is not None:
print("Data successfully scraped, creating summary prompt")
prompt = create_summary_prompt(scraped_data)
print("Sending prompt to ChatGPT for summarization")
model = ChatOpenAI(model="gpt-4o-mini")
response = model.invoke(prompt)
summary = response.content
print("Received summary from ChatGPT")
export_data = {
"url": article_url,
"summary": summary
}
file_name = "summary.json"
with open(file_name, "w") as file:
json.dump(export_data, file, indent=4)
print(f"Data exported to '{file_name}'")
else:
print("Scraping failed")
Run the script with:
python3 script.py
Or, on Windows:
python script.py
Open the summary.json file that appeared in the project’s directory to view your scraped data.
Using LangChain for Web Scraping: Why ProxyTee Makes the Difference
In this tutorial, you learned why web scraping is an excellent method for gathering data for your AI workflows and how to analyze it using LangChain, leveraging ProxyTee. Specifically, you learned how to create a Python-based LangChain web scraping script to extract data from a CNN news article and process it with OpenAI APIs.
The main challenges with web scraping include:
- Websites frequently change their page structures.
- Many sites implement advanced anti-bot measures.
- Retrieving large volumes of data simultaneously can be complex.
ProxyTee’s rotating residential proxies offers a solution for these challenges. This makes it a valuable tool for supporting RAG applications and other LangChain-powered solutions.
With features like unlimited bandwidth, over 20 million IPs across 100+ countries, and flexible geo-targeting options, ProxyTee ensures a reliable and scalable web scraping process. Plus, its competitive pricing and simple user interface makes it an ideal choice for individuals and businesses.
Be sure to explore our additional offerings for AI and LLM at Use cases. You can also start with a free trial to experience the benefits firsthand by clicking the pricing.